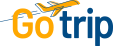
Convallis id congue eu quam
Mauris ullamcorper felis vitae
Augue non elementum
Lorem ipsum dolor sit amet, consectetur adipisicing elit, sed do eiusmod tempor incididunt ut labore et dolore magna aliqua. Ut enim ad minim veniam, quis nostrud
Integer quis metus vitae elit lobortis egestas. Lorem ipsum dolor sit amet, consectetuer adipiscing elit. Morbi vel erat non mauris convallis vehicula.
Aliquam
| Ligula massa
| Ligula massa